Nigeria
2024-12-26 03:58
IndustryQUANTITATIVE AND ALGORITHMIC TRADING
#ANNUALINVESTMENTSHARINGMICHRICHES#
Quantitative and Algorithmic Trading Strategies: An Overview
Quantitative and algorithmic trading have become central to modern financial markets, enabling traders to execute strategies that leverage vast amounts of data, mathematical models, and computational power to make decisions faster and more efficiently than traditional methods. Below is a breakdown of the key strategies, approaches, and concepts in this domain.
1. Quantitative Trading: An Overview
Quantitative trading, often referred to as “quant trading,” involves the use of mathematical models, statistical analysis, and historical data to identify trading opportunities. Quants typically design strategies based on price patterns, economic indicators, and market behavior. The aim is to create models that can predict future price movements or identify inefficiencies in the market that can be exploited for profit.
Key Features of Quantitative Trading:
• Data-Driven: Relies heavily on large datasets, including historical price data, financial statements, and macroeconomic indicators.
• Statistical Models: Uses complex mathematical and statistical models to identify trends, correlations, and potential market anomalies.
• Backtesting: Before being deployed, strategies are rigorously tested against historical data to evaluate their effectiveness.
• Risk Management: Incorporates advanced techniques like portfolio optimization and risk models (e.g., Value at Risk, drawdown measures) to minimize losses.
2. Algorithmic Trading: An Overview
Algorithmic trading refers to the use of computer algorithms to automate the process of buying and selling financial instruments, such as stocks, bonds, and derivatives. The algorithms are designed to execute trades based on predefined criteria such as price, volume, time, and other market conditions.
Key Features of Algorithmic Trading:
• Automation: Trades are executed automatically, without human intervention, based on predefined rules or signals.
• Speed: Algorithms can analyze vast amounts of market data and execute trades within milliseconds or microseconds.
• Market Impact Minimization: Algorithms are designed to minimize the market impact of large orders by breaking them into smaller parts, reducing the likelihood of slippage.
• High-Frequency Trading (HFT): A subset of algorithmic trading that involves executing many orders at extremely high speeds to exploit minute market inefficiencies.
3. Common Quantitative and Algorithmic Trading Strategies
a. Trend Following Strategies
• Concept: These strategies are based on the belief that assets that are trending (either up or down) will continue to move in the same direction for some time.
• Implementation: Common methods include moving averages (simple, exponential, or weighted), momentum indicators, and trend-following models such as Moving Average Convergence Divergence (MACD).
• Example: A simple strategy might be to buy an asset when its 50-day moving average crosses above its 200-day moving average (a “golden cross”) and sell when the reverse occurs (a “death cross”).
b. Mean Reversion Strategies
• Concept: Based on the idea that asset prices and returns eventually move back to their historical mean or average level.
• Implementation: Traders use statistical models like the Bollinger Bands, Z-score, or relative strength index (RSI) to identify overbought or oversold conditions.
• Example: A mean reversion strategy might involve buying an asset when its price falls more than two standard deviations below its 30-day moving average and selling when it rises above that threshold.
c. Statistical Arbitrage
• Concept: This strategy involves creating portfolios of long and short positions in related securities to exploit pricing inefficiencies between them.
• Implementation: Pairs trading is one of the most common methods, where two stocks that have historically moved in correlation are traded based on their relative price movements.
• Example: If Stock A is undervalued relative to Stock B (historically correlated), a trader might go long on Stock A and short Stock B, betting that the prices will converge.
d. Market Making
• Concept: Market makers provide liquidity to the market by continuously quoting both buy and sell prices for a particular asset. They profit from the bid-ask spread.
• Implementation: Algorithms monitor order books and make decisions based on order flow, supply/demand imbalances, and market conditions.
• Example: A market-making algorithm may place buy orders just below the current market price and sell orders just above, profiting from the difference between them.
e. Momentum Strategies
• Concept: These strategies are based on the observation that assets which have performed well in the past will continue to perform well in the future, and vice versa for underperforming.
Like 0
OLUWAPELUMI
Trader
Hot content
Industry
Event-A comment a day,Keep rewards worthy up to$27
Industry
Nigeria Event Giveaway-Win₦5000 Mobilephone Credit
Industry
Nigeria Event Giveaway-Win ₦2500 MobilePhoneCredit
Industry
South Africa Event-Come&Win 240ZAR Phone Credit
Industry
Nigeria Event-Discuss Forex&Win2500NGN PhoneCredit
Industry
[Nigeria Event]Discuss&win 2500 Naira Phone Credit
Forum category
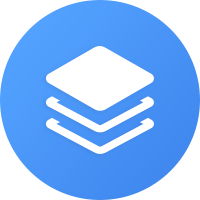
Platform
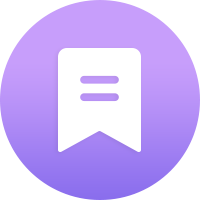
Exhibition
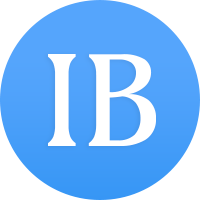
Agent
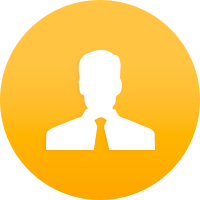
Recruitment
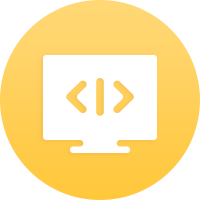
EA
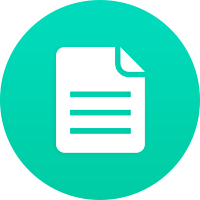
Industry
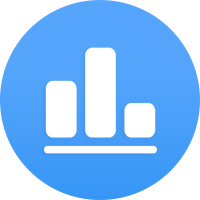
Market
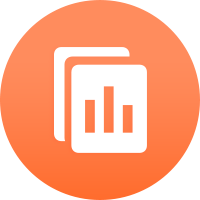
Index
QUANTITATIVE AND ALGORITHMIC TRADING
#ANNUALINVESTMENTSHARINGMICHRICHES#
Quantitative and Algorithmic Trading Strategies: An Overview
Quantitative and algorithmic trading have become central to modern financial markets, enabling traders to execute strategies that leverage vast amounts of data, mathematical models, and computational power to make decisions faster and more efficiently than traditional methods. Below is a breakdown of the key strategies, approaches, and concepts in this domain.
1. Quantitative Trading: An Overview
Quantitative trading, often referred to as “quant trading,” involves the use of mathematical models, statistical analysis, and historical data to identify trading opportunities. Quants typically design strategies based on price patterns, economic indicators, and market behavior. The aim is to create models that can predict future price movements or identify inefficiencies in the market that can be exploited for profit.
Key Features of Quantitative Trading:
• Data-Driven: Relies heavily on large datasets, including historical price data, financial statements, and macroeconomic indicators.
• Statistical Models: Uses complex mathematical and statistical models to identify trends, correlations, and potential market anomalies.
• Backtesting: Before being deployed, strategies are rigorously tested against historical data to evaluate their effectiveness.
• Risk Management: Incorporates advanced techniques like portfolio optimization and risk models (e.g., Value at Risk, drawdown measures) to minimize losses.
2. Algorithmic Trading: An Overview
Algorithmic trading refers to the use of computer algorithms to automate the process of buying and selling financial instruments, such as stocks, bonds, and derivatives. The algorithms are designed to execute trades based on predefined criteria such as price, volume, time, and other market conditions.
Key Features of Algorithmic Trading:
• Automation: Trades are executed automatically, without human intervention, based on predefined rules or signals.
• Speed: Algorithms can analyze vast amounts of market data and execute trades within milliseconds or microseconds.
• Market Impact Minimization: Algorithms are designed to minimize the market impact of large orders by breaking them into smaller parts, reducing the likelihood of slippage.
• High-Frequency Trading (HFT): A subset of algorithmic trading that involves executing many orders at extremely high speeds to exploit minute market inefficiencies.
3. Common Quantitative and Algorithmic Trading Strategies
a. Trend Following Strategies
• Concept: These strategies are based on the belief that assets that are trending (either up or down) will continue to move in the same direction for some time.
• Implementation: Common methods include moving averages (simple, exponential, or weighted), momentum indicators, and trend-following models such as Moving Average Convergence Divergence (MACD).
• Example: A simple strategy might be to buy an asset when its 50-day moving average crosses above its 200-day moving average (a “golden cross”) and sell when the reverse occurs (a “death cross”).
b. Mean Reversion Strategies
• Concept: Based on the idea that asset prices and returns eventually move back to their historical mean or average level.
• Implementation: Traders use statistical models like the Bollinger Bands, Z-score, or relative strength index (RSI) to identify overbought or oversold conditions.
• Example: A mean reversion strategy might involve buying an asset when its price falls more than two standard deviations below its 30-day moving average and selling when it rises above that threshold.
c. Statistical Arbitrage
• Concept: This strategy involves creating portfolios of long and short positions in related securities to exploit pricing inefficiencies between them.
• Implementation: Pairs trading is one of the most common methods, where two stocks that have historically moved in correlation are traded based on their relative price movements.
• Example: If Stock A is undervalued relative to Stock B (historically correlated), a trader might go long on Stock A and short Stock B, betting that the prices will converge.
d. Market Making
• Concept: Market makers provide liquidity to the market by continuously quoting both buy and sell prices for a particular asset. They profit from the bid-ask spread.
• Implementation: Algorithms monitor order books and make decisions based on order flow, supply/demand imbalances, and market conditions.
• Example: A market-making algorithm may place buy orders just below the current market price and sell orders just above, profiting from the difference between them.
e. Momentum Strategies
• Concept: These strategies are based on the observation that assets which have performed well in the past will continue to perform well in the future, and vice versa for underperforming.
Like 0
I want to comment, too
Submit
0Comments
There is no comment yet. Make the first one.
Submit
There is no comment yet. Make the first one.