Malaysia
2025-05-07 10:13
IndustryAdaptive signal prioritization inforex via Al
#AIImpactOnForex
AI can significantly enhance adaptive signal prioritization in Forex trading by dynamically adjusting the importance or weight given to various trading signals based on real-time market conditions and the historical performance of those signals. Here's a detailed explanation:
1. Dynamic Weighting of Signals:
* AI algorithms can analyze a multitude of technical and fundamental signals, such as moving averages, RSI, MACD, Fibonacci levels, volatility indicators, and even news sentiment.
* Instead of using fixed weights for each signal, AI models can learn the optimal weighting for each signal based on the current market regime. For instance, in a trending market, trend-following signals might be given higher priority, while in a ranging market, signals from oscillators or support and resistance levels could be emphasized.
* Machine learning techniques, like neural networks or reinforcement learning, can continuously evaluate the profitability and reliability of different signals under various market conditions and adjust their weights accordingly.
2. Contextual Awareness:
* AI can consider the broader market context when prioritizing signals. This includes analyzing the strength and consistency of the overall trend, market volatility, and even the time of day.
* For example, a strong buy signal appearing during a period of high market volatility might be given a lower priority compared to the same signal occurring in a more stable environment, as the likelihood of false breakouts increases during volatile periods.
3. Individualized Prioritization:
* AI can personalize signal prioritization based on a trader's risk tolerance, trading style, and preferred currency pairs.
* A risk-averse trader might prefer signals with a higher historical win rate, even if they occur less frequently, while a more aggressive trader might opt for higher-frequency signals, even with slightly lower confidence levels. AI can learn these preferences and adjust the signal weighting accordingly.
4. Real-time Adaptation:
* The Forex market is highly dynamic. AI algorithms can continuously monitor the performance of prioritized signals in real-time and adapt the weighting as market conditions evolve.
* If a previously high-priority signal starts to generate more losing trades under new market conditions, the AI can automatically reduce its weight and increase the importance of more profitable signals.
5. Ensemble Methods:
* AI can employ ensemble learning techniques by combining multiple AI models, each trained to prioritize signals differently. The final signal prioritization can be a weighted average of the prioritizations from individual models, potentially leading to more robust and adaptive decision-making.
General Information and Useful Details:
* Types of AI Models: Various AI models can be used for adaptive signal prioritization, including:
* Neural Networks: Capable of learning complex non-linear relationships between signals and market outcomes.
* Decision Trees and Random Forests: Can identify important signals and their interaction effects.
* Reinforcement Learning: Algorithms that learn through trial and error, optimizing signal weights based on the profitability of resulting trades.
* Time Series Analysis (ARIMA, LSTMs): Can analyze the sequential nature of signals and market data to predict future signal reliability.
* Feature Engineering: The selection and engineering of relevant features (the signals themselves and their historical performance metrics) are crucial for the success of AI-driven signal prioritization.
* Backtesting and Validation: Any AI-based signal prioritization strategy must be rigorously backtested on historical data and validated on live or simulated trading environments to ensure its effectiveness and robustness.
* Integration with Trading Platforms: AI-powered adaptive signal prioritization can be integrated with existing Forex trading platforms through APIs or custom-developed tools, providing traders with dynamic and intelligent trading guidance.
* Risk Management: Adaptive signal prioritization should always be coupled with sound risk management strategies, such as setting stop-loss orders and managing position sizes, to protect capital.
In essence, AI offers a sophisticated approach to Forex trading by moving beyond static signal analysis to a dynamic and adaptive system that learns from market behavior and trader preferences to prioritize the most relevant and potentially profitable trading signals in real-time.
Like 0
niki845
Trader
Hot content
Industry
Event-A comment a day,Keep rewards worthy up to$27
Industry
Nigeria Event Giveaway-Win₦5000 Mobilephone Credit
Industry
Nigeria Event Giveaway-Win ₦2500 MobilePhoneCredit
Industry
South Africa Event-Come&Win 240ZAR Phone Credit
Industry
Nigeria Event-Discuss Forex&Win2500NGN PhoneCredit
Industry
[Nigeria Event]Discuss&win 2500 Naira Phone Credit
Forum category
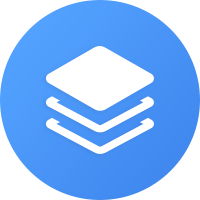
Platform
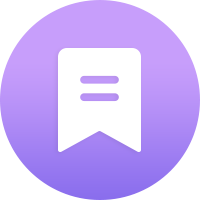
Exhibition
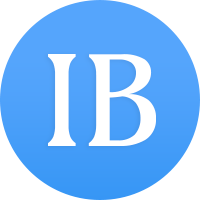
Agent
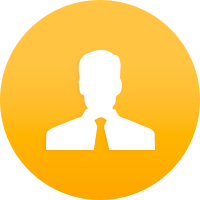
Recruitment
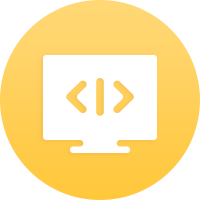
EA
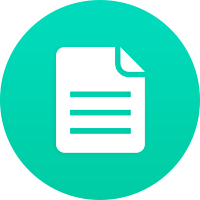
Industry
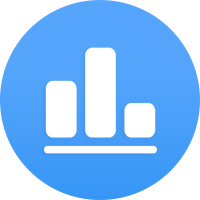
Market
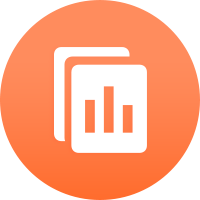
Index
Adaptive signal prioritization inforex via Al
#AIImpactOnForex
AI can significantly enhance adaptive signal prioritization in Forex trading by dynamically adjusting the importance or weight given to various trading signals based on real-time market conditions and the historical performance of those signals. Here's a detailed explanation:
1. Dynamic Weighting of Signals:
* AI algorithms can analyze a multitude of technical and fundamental signals, such as moving averages, RSI, MACD, Fibonacci levels, volatility indicators, and even news sentiment.
* Instead of using fixed weights for each signal, AI models can learn the optimal weighting for each signal based on the current market regime. For instance, in a trending market, trend-following signals might be given higher priority, while in a ranging market, signals from oscillators or support and resistance levels could be emphasized.
* Machine learning techniques, like neural networks or reinforcement learning, can continuously evaluate the profitability and reliability of different signals under various market conditions and adjust their weights accordingly.
2. Contextual Awareness:
* AI can consider the broader market context when prioritizing signals. This includes analyzing the strength and consistency of the overall trend, market volatility, and even the time of day.
* For example, a strong buy signal appearing during a period of high market volatility might be given a lower priority compared to the same signal occurring in a more stable environment, as the likelihood of false breakouts increases during volatile periods.
3. Individualized Prioritization:
* AI can personalize signal prioritization based on a trader's risk tolerance, trading style, and preferred currency pairs.
* A risk-averse trader might prefer signals with a higher historical win rate, even if they occur less frequently, while a more aggressive trader might opt for higher-frequency signals, even with slightly lower confidence levels. AI can learn these preferences and adjust the signal weighting accordingly.
4. Real-time Adaptation:
* The Forex market is highly dynamic. AI algorithms can continuously monitor the performance of prioritized signals in real-time and adapt the weighting as market conditions evolve.
* If a previously high-priority signal starts to generate more losing trades under new market conditions, the AI can automatically reduce its weight and increase the importance of more profitable signals.
5. Ensemble Methods:
* AI can employ ensemble learning techniques by combining multiple AI models, each trained to prioritize signals differently. The final signal prioritization can be a weighted average of the prioritizations from individual models, potentially leading to more robust and adaptive decision-making.
General Information and Useful Details:
* Types of AI Models: Various AI models can be used for adaptive signal prioritization, including:
* Neural Networks: Capable of learning complex non-linear relationships between signals and market outcomes.
* Decision Trees and Random Forests: Can identify important signals and their interaction effects.
* Reinforcement Learning: Algorithms that learn through trial and error, optimizing signal weights based on the profitability of resulting trades.
* Time Series Analysis (ARIMA, LSTMs): Can analyze the sequential nature of signals and market data to predict future signal reliability.
* Feature Engineering: The selection and engineering of relevant features (the signals themselves and their historical performance metrics) are crucial for the success of AI-driven signal prioritization.
* Backtesting and Validation: Any AI-based signal prioritization strategy must be rigorously backtested on historical data and validated on live or simulated trading environments to ensure its effectiveness and robustness.
* Integration with Trading Platforms: AI-powered adaptive signal prioritization can be integrated with existing Forex trading platforms through APIs or custom-developed tools, providing traders with dynamic and intelligent trading guidance.
* Risk Management: Adaptive signal prioritization should always be coupled with sound risk management strategies, such as setting stop-loss orders and managing position sizes, to protect capital.
In essence, AI offers a sophisticated approach to Forex trading by moving beyond static signal analysis to a dynamic and adaptive system that learns from market behavior and trader preferences to prioritize the most relevant and potentially profitable trading signals in real-time.
Like 0
I want to comment, too
Submit
0Comments
There is no comment yet. Make the first one.
Submit
There is no comment yet. Make the first one.